Ethereum
A Concept of Collective aI on Ethereum and Ethereum Swarm
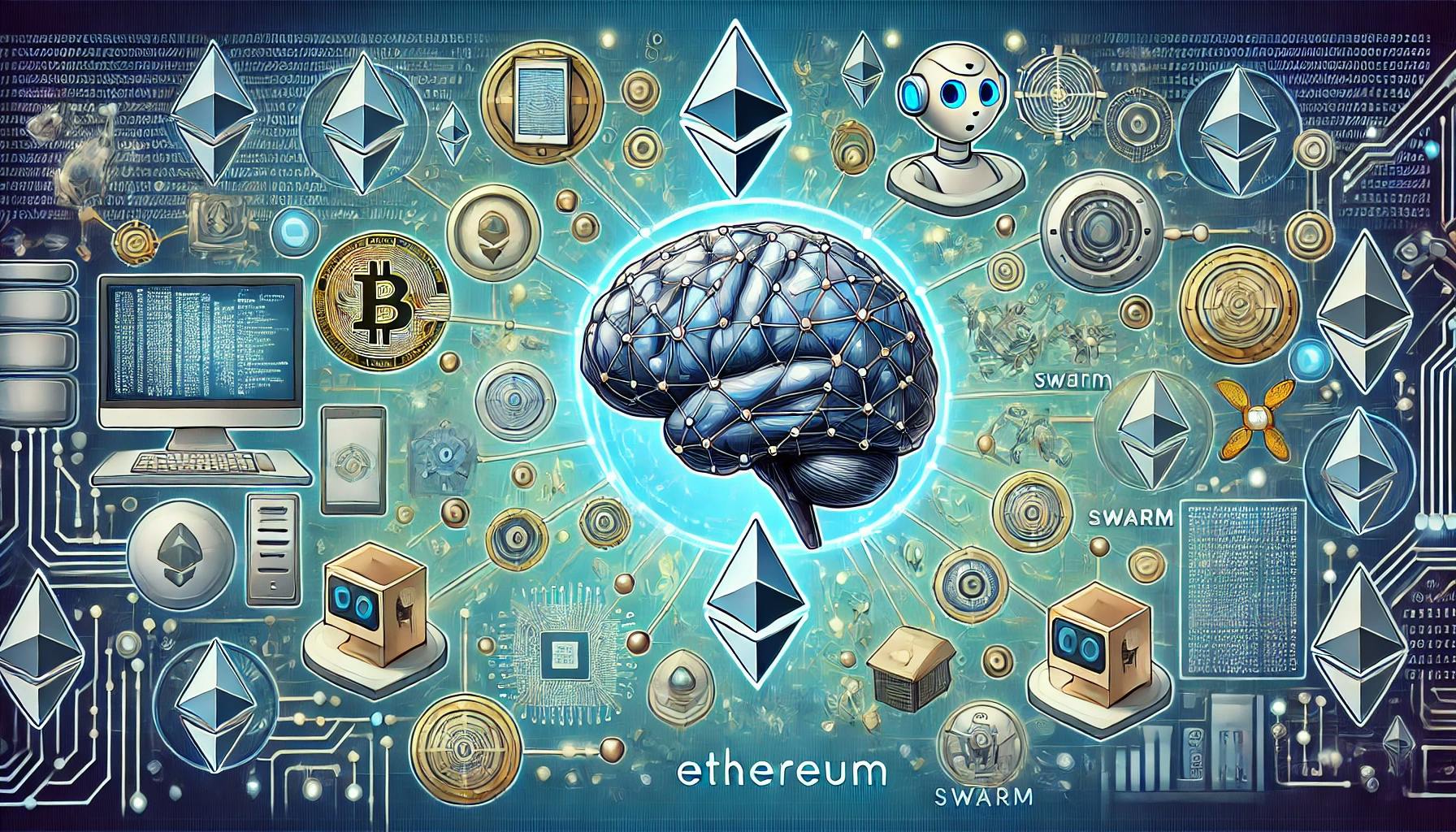
Currently, the key players in AI can be divided into two major groups: supporters of open-source AI and supporters of closed AI.
Interestingly, one of the biggest supporters of closed AI is OpenAI itself, which does not release the source code of its models, only provides access to them. They usually argue that it would be too dangerous to publish these models, thus centralized control is necessary, just like with nuclear energy. Obviously, there is a basis for this argument, but it is not hard to see the business interests behind the decision. If the source code of ChatGPT were available to everyone, who would pay for the service?!
In contrast, supporters of open-source AI, such as Meta (Facebook), believe that closed AI hinders progress and that open-source AI is the right direction. Of course, it is also worth seeing the business aspects here. For Meta, the AI model is not the main product. For them, AI is just a tool, and sharing the model does not pose a business disadvantage. On the contrary, it provides a business advantage, as Meta can later utilize the community’s developments. However, there is a small problem with this model as well. It is not truly open-source.
An AI model is essentially a huge mathematical equation with adjustable parameters. These parameters are set during the training process. Whenever a company talks about open-source AI, it means that these parameters are made freely accessible so that anyone can run the model on their machine. But it is not fully open-source!
In the case of AI, training is analogous to building in traditional programs. Based on this, the model parameters represent the binary file. So when Meta, X (Twitter), or other companies make their model source open, they are actually just giving away the result.
So what we get is a fixed architecture’s parameterization. If we want to change or improve anything in the architecture, for example, use a Mamba architecture instead of a Transformer architecture, we would need to retrain the model, which we cannot do without the training set. Therefore, these models can only be fine-tuned, not further developed.
The so-called open-source models are not truly open-source, as the architecture is fixed. These models can only be fine-tuned but not further developed, as that would require the training set as well. True open-source AI consists of both the model and the training set!
“Open-source” AI models are typically products of large companies. This is understandable, as training a large model requires a tremendous amount of computational capacity and, consequently, a lot of money. Only big companies have such resources, which is why AI development is centralized.
Just as blockchain technology in the form of Bitcoin created the possibility of decentralized money, it also allows us to create truly open-source AI that is owned by the community instead of a company.
This article is a concept on how such a truly open-source, community-driven AI could be developed using blockchain technology.
As I mentioned earlier, the foundation of a truly open-source AI is an open dataset. The dataset is actually the most valuable resource. In the case of ChatGPT, for example, the language model was trained on publicly available databases (e.g., Common Crawl), and then fine-tuned with human assistance (RLHF) in a subsequent phase. This fine-tuning is extremely costly due to the human labor involved, but it is what gives ChatGPT its strength. The architecture itself is (presumably) a general transformer or a modified version of it, the Mixture of Experts, which means multiple parallel transformers. The key point is that the architecture is not special. What makes ChatGPT (and every other model) unique is the good dataset. This is what gives the model its power.
An AI training dataset is typically several terabytes in size, and what can or cannot be included in such a dataset can vary by group and culture. The choice of data is very important, as it will determine, for example, the ‘personality’ of a large language model. Several major scandals have erupted because AI models from big companies (Google, Microsoft, etc.) behaved in a racist manner. This is due to the improper selection of the dataset. Since the requirements for the dataset can vary by culture, multiple forks may be necessary. Decentralized, content-addressed storage solutions like IPFS or Ethereum Swarm are ideal for storing such versioned, multi-fork large datasets. These storage solutions work similarly to the GIT version control system, where individual files can be addressed with a hash generated from the content. In such systems, forks can be created cheaply because only the changes need to be stored, and the common part of the two datasets is stored in a single instance.
Once we have the appropriate datasets, we can proceed with training the model.
As mentioned in the introduction, an AI model is essentially a gigantic mathematical equation with numerous free parameters. It is generally true that the more free parameters a model has, the ‘smarter’ it is, so the number of parameters is often indicated in the model’s name. For example, the llma-2-7b model means that the model architecture is llma-2 and has 7 billion parameters. During training, these parameters are set using the dataset so that the model provides the specified output for the given input. Backpropagation is used for training, which finds the most fitting parameters with the help of partial derivatives.
During training, the dataset is divided into batches. In each step, a given batch provides the input and output parameters, and backpropagation is used to calculate how the model’s parameters need to be modified to accurately compute the given output from the given input. This process must be repeated multiple times on the given dataset until the model achieves the desired accuracy. The accuracy can be checked with the test dataset.
Large companies conduct training on massive GPU clusters because training requires enormous computational capacity. In a decentralized system, an additional challenge is that individual nodes are unreliable, and there is always a cost associated with unreliability! This unreliability is why Bitcoin has the energy consumption of a small country. Bitcoin uses Proof of Work consensus, where computational capacity replaces reliability. Instead of trusting individual nodes, we trust that well-intentioned nodes possess more computational capacity than malicious ones in the network. Fortunately, there are other consensus mechanisms, such as Proof of Stake used by Ethereum, where staked money guarantees our reliability instead of computational capacity. In this case, there is no need for large computational capacity, resulting in significantly lower energy demand and environmental impact.
In decentralized training, some mechanism is needed to replace the trust between the training node and the requester. One possible solution is for the training node to create a log of the entire training process, and a third party, a validator node, randomly checks the log at certain points. If the validator node finds the training satisfactory, the training node receives the offered payment. The validator cannot check the entire log, as that would mean redoing all the computations, and the validation’s computational requirements would equal those of the training.
Another option is the optimistic solution, where we assume that the node performed the computation correctly and provide a challenge period during which anyone can prove otherwise. In this case, the node performing the computation stakes a larger amount (penalty), and the node requesting the computation also stakes an amount (reward). The node performs the computation and then publishes the result. This is followed by the challenge period (for example, 1 day). If someone finds an error in the computation with random checks during this period and publishes it, they receive the penalty staked by the computing node, and the requester gets their reward back. If no one can prove that the computation is incorrect during the challenge period, the computing node receives the reward.
There is a variant of zero-knowledge proofs called zkSNARK, which is also suitable for verifying that someone has performed a computation. The main advantage of this method is that the verification can be done cheaply, but generating the proof is a computationally intensive task. Since this method is very costly even for simpler computations, it would require significantly more computational resources for AI training than the training itself, so we probably cannot use it for this purpose at present. Nevertheless, zkML is an active research area, and it is conceivable that in the future, the third party could be replaced by a smart contract that verifies the SNARK.
From the above, it is clear that there are several solutions for verifying computations. Based on these, let’s see how our blockchain-based decentralized training support system would be built.
In this system, datasets are owned by the community through DAOs. The DAO decides what data can be included in the dataset. If a group of members disagrees with the decision, they can split from the DAO and form a new DAO, where they fork the existing dataset and continue to build it independently. Thus, the DAO is forked along with the dataset. Since the dataset is stored in content-addressed decentralized storage (e.g., Ethereum Swarm), forking is not expensive. The storage of the dataset is financed by the community.
The training process is also controlled by a DAO. Through the DAO, training nodes that wish to sell their spare computational capacity can register. To apply, they must place a stake in a smart contract. If a node attempts to cheat during the computation, it will lose this stake.
The requester selects the dataset and the model they want to train and then offers a reward. The offer is public, so any training node can apply to perform the task. The training node creates a complete log of the training process, where each entry corresponds to the training of a batch. The entry includes the input, the output, the weight matrix, and all relevant parameters (e.g., the random seed used by the dropout layer to select the data to be dropped). Thus, the entire computation can be reproduced based on the log.
As mentioned earlier, several methods can be used to verify the computation. The simplest is the optimistic approach. In this case, the requester places the reward in a smart contract, and the training node publishes the training log. After the publication, a specified time frame (e.g., 1 day) is available for verifying the computation. If during this time the requester or anyone else submits proof that a particular step is incorrect, the training node loses its stake, and the requester gets the reward back. In this case, the node who submits the correct proof receives the stake, incentivizing everyone to validate the computations. If no one submits such proof, the training node receives the reward after the time expires.
In a nutshell, this is how the system works. Of course, a few questions arise.
Who will pay for the cost of training and storing the datasets?
The business model of the system is the same as most free and open-source solutions, such as the Linux business model. If a company needs a model and has no problem with it being free and open-source, it is much more cost-effective to invest in this than to train its own model. Imagine that 10 companies need the same language model. If they don’t mind the model being open, it’s much more economical for each to pay 1/10th of the training cost rather than each paying the full amount. The same applies to the datasets that form the basis for training. Crowdfunding campaigns can even be created for training models, where future users of the model can contribute to its development.
Isn’t it cheaper to train models in the cloud?
Since prices in such a system are regulated by the market, it is difficult to give a definitive answer to this. It depends on how much free computational capacity is available to users. We have already seen the power of the community with Bitcoin. The computational capacity of the Bitcoin network surpasses that of any supercomputer. Cloud providers need to generate profit, whereas in a decentralized system like this, users offer their spare computational capacity. For example, someone with a powerful gaming PC can offer their spare capacity when they are not playing. In this case, if the service generates slightly more than the energy used, it is already worthwhile for the user. Additionally, there is a lot of waste energy in the world that cannot be utilized through traditional means. An example of this is the thermal energy produced by volcanoes. These locations typically do not have an established electrical grid, making them unsuitable for generating usable electricity. There are already startups using this energy for Bitcoin mining. Why not use it for ‘intelligence mining’? Since the energy in this case is virtually free, only the cost of the hardware needs to be covered. Thus, it is evident that there are many factors that could make training in such a decentralized system much cheaper than in the cloud.
What about inference?
In the case of running AI models, privacy is a very important issue. Large service providers naturally guarantee that they handle our data confidentially, but can we be sure that no one is eavesdropping on our conversations with ChatGPT? There are methods (e.g., homomorphic encryption) that allow servers to perform computations on encrypted data, but these have high overheads. The most secure solution is to run the models locally. Fortunately, hardware is getting stronger, and there are already specialized hardware solutions for running AI. The models themselves are also improving significantly. Research shows that in many cases, performance does not degrade much even after quantization, even in extreme cases where only 1.5 bits are used to represent weights. This latter solution is particularly promising because it eliminates multiplication, which is the most costly operation. Thus, in the future, thanks to the development of models and hardware, we are likely to run models that exceed human level locally. Moreover, we can customize these models to our liking with solutions like LoRA.
Distributed knowledge
Another very promising direction is retrieval-augmented generation (RAG). This means that ‘lexical knowledge’ is stored in a vector database, and our language model gathers the appropriate context from this database for the given question. This is very similar to how we humans function. Clearly, no one memorizes an entire lexicon. When asked a question, it’s enough to know where to find the necessary knowledge. By reading and interpreting the relevant entries, we can provide a coherent answer. This solution has numerous advantages. On one hand, a smaller model is sufficient, which is easier to run locally, and on the other hand, hallucination, a major problem with language models, can be minimized. Additionally, the model’s knowledge can be easily expanded without retraining, simply by adding new knowledge to the vector database. Ethereum Swarm is an ideal solution for creating such a vector database, as it is not only a decentralized storage engine but also a communication solution. For example, group messaging can be implemented over Swarm, enabling the creation of a simple distributed vector database. The node publishes the search query, and the other nodes respond by returning the related knowledge.
Summary: Implementation of LLM OS over Ethereum and Swarm
The idea of LLM OS originates from Andrej Karpathy, which he published on Twitter. LLM OS is a hypothetical operating system centered around a large language model. In our blockchain-based distributed system, we can consider this as an agent running on a user’s node. This agent can communicate with other agents and traditional Software 1.0 tools. These can include a calculator, a Python interpreter, or even control a physical robot, car, or smart home. In our system, the file system is represented by Swarm and the vector database created over Swarm, where common knowledge is accessible. The entire system (the collective of agents) can be viewed as a form of collective intelligence.
I believe that in the future, artificial intelligence will become a part of our daily lives, much more integrally than it is now. AI will become a part of us! Instead of mobile phones, we will wear smart glasses with cameras that record everything and microphones that hear everything. We will have continuous dialogues with our locally running language models and other agents, which will adapt to our needs over time through fine-tuning. But these agents will not only communicate with us but also with each other, constantly utilizing the collective knowledge produced by the entire community. This system will organize humanity into a form of collective intelligence, which is a very significant thing. It is not acceptable for this collective intelligence to become the property of a single company or entity. That is why we need the systems outlined above, or similar ones!
Ethereum
QCP sees Ethereum as a safe bet amid Bitcoin stagnation
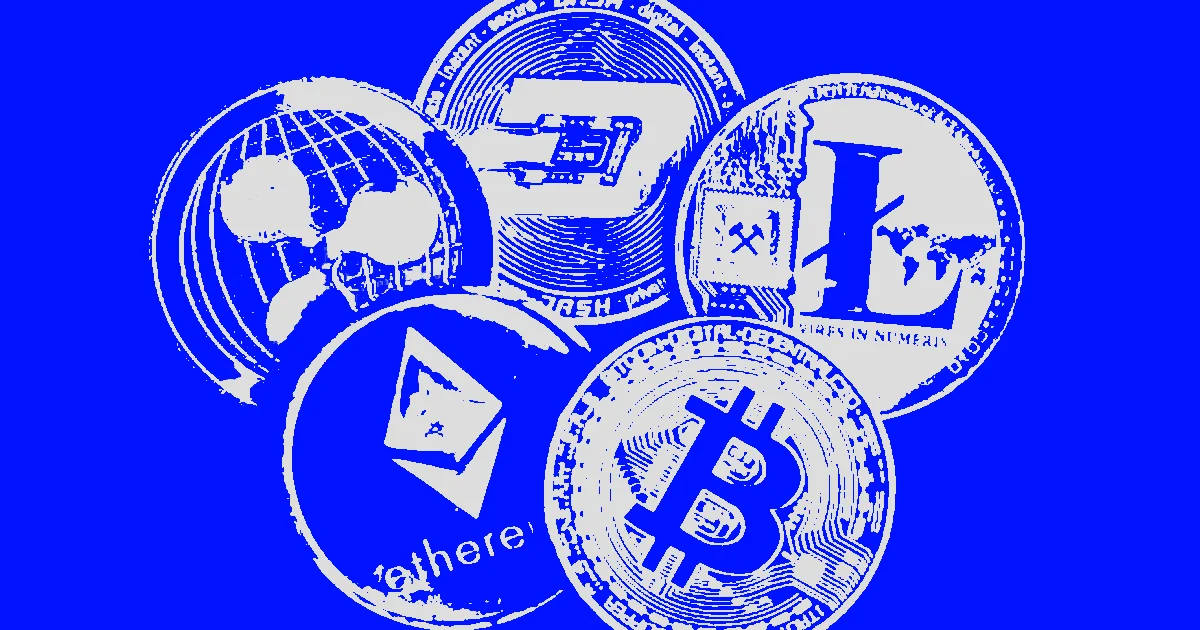
QCP, a leading trading firm, has shared key observations on the cryptocurrency market. Bitcoin’s struggle to surpass the $70,000 mark has led QCP to predict Selling pressure is still strong, with BTC likely to remain in a tight trading range. In the meantime, Ethereum (ETH) is seen as a more promising investment, with potential gains as ETH could catch up to BTC, thanks to decreasing ETHE outflows.
Read on to find out how you can benefit from it.
Bitcoin’s Struggle: The $70,000 Barrier
For the sixth time in a row, BTC has failed to break above the $70,000 mark. Bitcoin is at $66,048 after a sharp decline. Many investors sold Bitcoin to capitalize on the rising values, which caused a dramatic drop. The market is becoming increasingly skeptical about Bitcoin’s rise, with some investors lowering their expectations.
Despite the continued sell-off from Mt. Gox and the US government, the ETF market remains bullish. There is a notable trend in favor of Ethereum (ETH) ETFs as major bulls have started investing in ETFs, indicating a bullish sentiment for ETH.
QCP Telegram Update UnderlinesIncreased market volatility. The NASDAQ has fallen 10% from its peak, led by a pullback in major technology stocks. Currency carry trades are being unwound and the VIX, a measure of market volatility, has jumped to 19.50.
The main factors driving this uncertainty are Value at Risk (VaR) shocks, high stock market valuations and global risk aversion sentiment. Commodities such as oil and copper have also declined on fears of an economic slowdown.
Additionally, QCP anticipates increased market volatility ahead of the upcoming FOMC meeting, highlighting the importance of the Federal Reserve’s statement and Jerome Powell’s subsequent press conference.
A glimmer of hope
QCP notes a positive development in the crypto space with an inflow of $33.7 million into ETH spot ETFs, which is giving a much-needed boost to ETH prices. However, they anticipate continued outflows of ETHE in the coming weeks. The recent Silk Road BTC moves by the US government have added to the market uncertainty.
QCP suggests a strategic trade involving BTC, which will likely remain in its current range, while ETH offers a more promising opportunity. They propose a trade targeting a $4,000-$4,500 range for ETH, which could generate a 5.5x return by August 30, 2024.
Ethereum
Ethereum Whale Resurfaces After 9 Years, Moves 1,111 ETH Worth $3.7 Million
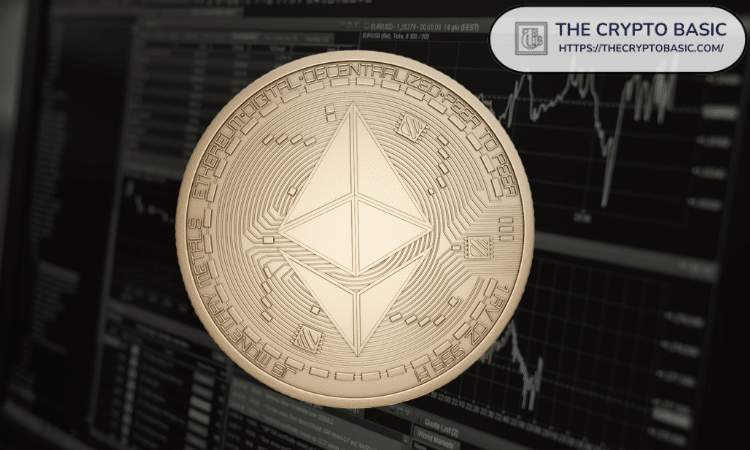
An Ethereum ICO participant has emerged from nearly a decade of inactivity.
Lookonchain, a smart on-chain money tracking tool, revealed On X, this long-inactive participant recently transferred 1,111 ETH, worth approximately $3.7 million, to a new wallet. This significant move marks a notable on-chain movement, given the participant’s prolonged dormancy.
The Ethereum account in question, identified as 0xE727E67E…B02B5bFC6, received 2,000 ETH on the Genesis block over 9 years ago.
This initial allocation took place during the Ethereum ICOwhere the participant invested in ETH at around $0.31 per coin. The initial investment, worth around $620 at the time, has now grown to millions of dollars.
Recent Transactions and Movements
The inactive account became active again with several notable output transactions. Specifically, the account transferred 1,000 ETH, 100 ETH, 10 ETH, 1 ETH, and 1 more ETH to address 0x7C21775C…2E9dCaE28 within a few minutes. Additionally, it moved 1 ETH to 0x2aa31476…f5aaCE9B.
Additionally, in the latest round of transactions, the address transferred 737,995 ETH, 50 ETH, and 100 ETH, for a total of 887,995 ETH. These recent activities highlight a significant movement of funds, sparking interest and speculation in the crypto community.
Why are whales reactivating?
It is also evident that apart from 0xE727E67E…B02B5bFC6, other previously dormant Ethereum whales are waking up with significant transfers.
In May, another dormant Ethereum whale made headlines when it staked 4,032 ETHvalued at $7.4 million, after more than two years of inactivity. This whale initially acquired 60,000 ETH during the Genesis block of Ethereum’s mainnet in 2015.
At the time, this activity could have been related to Ethereum’s upgrade known as “Shanghai,” which improved the network’s scalability and performance. This whale likely intended to capitalize on the price surge that occurred after the upgrade.
Disclaimer: This content is informational and should not be considered financial advice. The opinions expressed in this article may include the personal opinions of the author and do not reflect the opinion of The Crypto Basic. Readers are encouraged to conduct thorough research before making any investment decisions. The Crypto Basic is not responsible for any financial losses.
-Advertisement-
Ethereum
Only Bitcoin and Ethereum are viable for ETFs in the near future
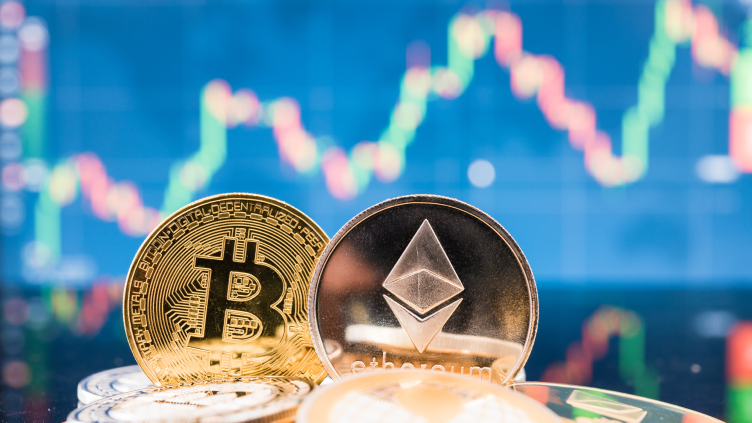
BlackRock: Only Bitcoin and Ethereum Are Viable for ETFs in the Near Future
Bitcoin and Ethereum will be the only cryptocurrencies traded via ETFs in the near future, according to Samara Cohen, chief investment officer of ETFs and indices at BlackRock, the world’s largest asset manager.
In an interview with Bloomberg TV, Cohen explained that while Bitcoin and Ethereum have met BlackRock’s rigorous criteria for exchange-traded funds (ETFs), no other digital asset currently comes close. “We’re really looking at the investability to see what meets the criteria, what meets the criteria that we want to achieve in an ETF,” Cohen said. “Both in terms of the investability and from what we’re hearing from our clients, Bitcoin and Ethereum definitely meet those criteria, but it’s going to be a while before we see anything else.”
Cohen noted that beyond the technical challenges of launching new ETFs, the demand for other crypto ETFs, particularly Solana, is not there yet. While Solana is being touted as the next potential ETF candidate, Cohen noted that the market appetite remains lacking.
BlackRock’s interest in Bitcoin and Ethereum ETFs comes after the successful launch of Ethereum ETFs last week, which saw weekly trading volume for the crypto fund soar to $14.8 billion, the highest level since May. The success has fueled speculation about the next possible ETF, with Solana frequently mentioned as a contender.
Solana, known as a faster and cheaper alternative to Ethereum, has been the subject of two separate ETF filings in the US by VanEck and 21Shares. However, the lack of CME Solana futures, unlike Bitcoin and Ethereum, is a significant hurdle for SEC approval of a Solana ETF.
Despite these challenges, some fund managers remain optimistic about Solana’s potential. Franklin Templeton recently described Solana as an “exciting and major development that we believe will drive the crypto space forward.” Solana currently accounts for about 3% of the overall cryptocurrency market value, with a market cap of $82 billion, according to data from CoinGecko.
Meanwhile, Bitcoin investors continue to show strong support, as evidenced by substantial inflows into BlackRock’s iShares Bitcoin Trust (NASDAQ: IBIT). On July 22, IBIT reported inflows of $526.7 million, the highest single-day total since March. This impressive haul stands in stark contrast to the collective inflow of just $6.9 million seen across the remaining 10 Bitcoin ETFs, according to data from Farside Investors. The surge in IBIT inflows coincides with Bitcoin’s significant $68,000 level, just 8% off its all-time high of $73,000.
Ethereum
Ethereum Posts First Consecutive Monthly Losses Since August 2023 on New ETFs
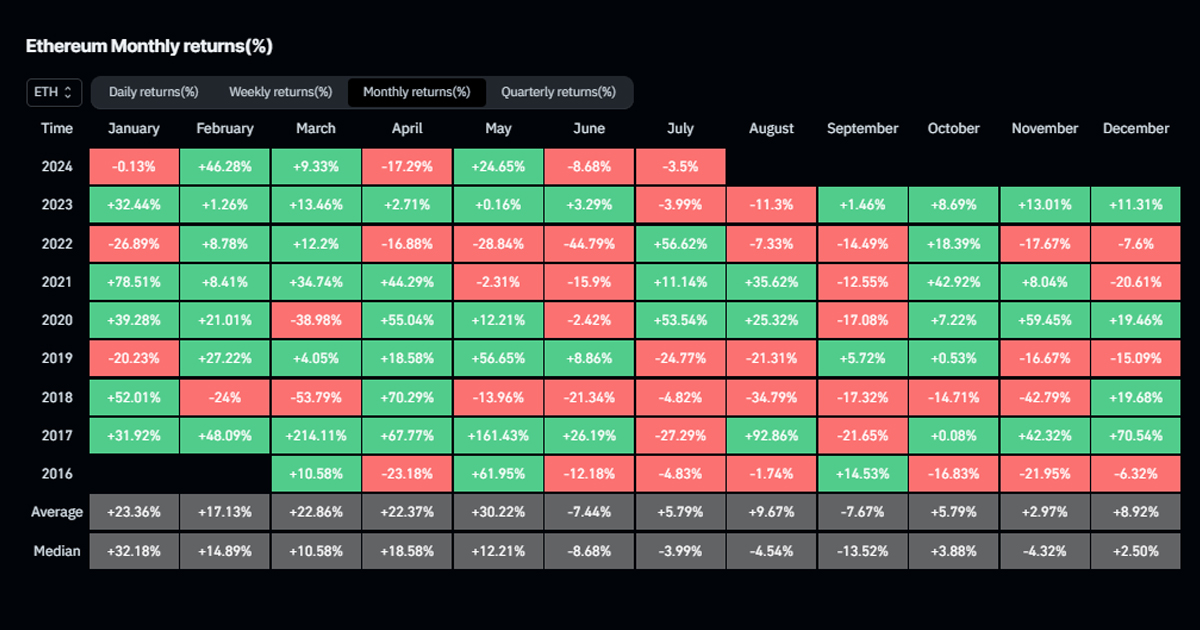
Available exclusively via
Bitcoin ETF vs Ethereum: A Detailed Comparison of IBIT and ETHA
Andjela Radmilac · 3 days ago
CryptoSlate’s latest market report takes an in-depth look at the technical and practical differences between IBIT and BlackRock’s ETHA to explain how these products work.
-
Ethereum10 months ago
Ethereum Posts First Consecutive Monthly Losses Since August 2023 on New ETFs
-
Regulation10 months ago
Cryptocurrency Regulation in Slovenia 2024
-
News10 months ago
New bill pushes Department of Veterans Affairs to examine how blockchain can improve its work
-
Regulation10 months ago
Think You Own Your Crypto? New UK Law Would Ensure It – DL News
-
Regulation10 months ago
A Blank Slate for Cryptocurrencies: Kamala Harris’ Regulatory Opportunity
-
Regulation10 months ago
Upbit, Coinone, Bithumb Face New Fees Under South Korea’s Cryptocurrency Law
-
Regulation10 months ago
Bahamas Passes Cryptocurrency Bill Designed to Prevent FTX, Terra Disasters
-
Regulation10 months ago
India to Follow G20 Policy for Cryptocurrency Regulation: MoS Finance
-
Ethereum12 months ago
Comment deux frères auraient dérobé 25 millions de dollars lors d’un braquage d’Ethereum de 12 secondes • The Register
-
Videos1 year ago
Nexus Chain – Ethereum L2 with the GREATEST Potential?
-
Ethereum1 year ago
Scaling Ethereum with L2s damaged its Tokenomics. Is it possible to repair it?
-
News1 year ago
Solana ranks the fastest blockchain in the world, surpassing Ethereum, Polygon ⋆ ZyCrypto